- The widespread adoption of generative AI (GenAI), exemplified by tools such as ChatGPT, is ushering society into a new era with novel risks and opportunities.
- The next phase of GenAI’s growth is a shift from training to inference, which could lead to soaring demand for computing infrastructure, from semiconductors to networking hardware, and data storage.
- Investors interested in tapping into the AI mega force may consider funds that seek to generate alpha through research-driven active equity management, and those that track thematic indexes encompassing the entire value chain of a theme.
How to invest for the next phase of AI
Sep 26, 2023 Equity
KEY TAKEAWAYS
WHAT IS GENERATIVE AI?
Generative artificial intelligence (AI) involves algorithms such as ChatGPT, designed to create diverse content like text, images, audio, code, and videos. Recent advancements in this field have the potential to revolutionize content generation, research methodologies, and beyond, opening doors to transformative opportunities. Achieving this and meeting continued future demand, however, will require substantial computing infrastructure and power.
THE NEW TECH STACK
In technology, software and hardware are pieced together to execute specific tasks or functions. This layered combination is referred to as a “stack” in the technology community. We believe that the future will require a brand-new stack to support the explosive growth of GenAI, with an increasing emphasis on specific types of semiconductors, super computers, progressively advanced models, novel approaches for managing data, and new applications for humans to leverage AI systems.
Visualizing the stack
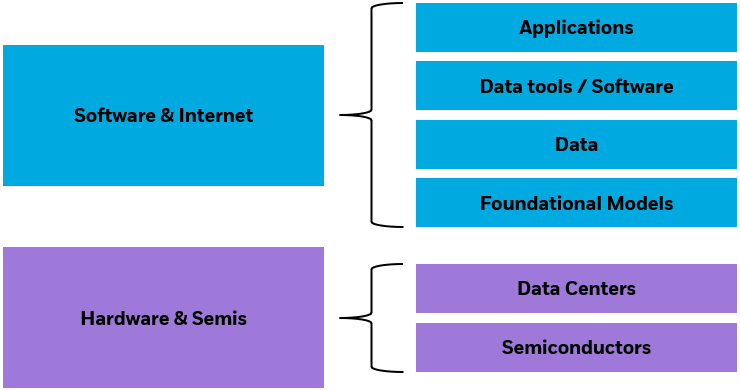
For illustrative purposes only. Subject to change.
Chart description: Flowchart showing the interaction between Software & Internet and Hardware & Semiconductors functions as the basis for performing various technological tasks — also known as a stack, shown visually above. As Generative AI gains momentum, a new stack is imperative to support its rapid expansion.
INFRASTRUCTURE UPGRADES NEEDED
As momentum around GenAI technology has accelerated this year, it has become clear that the physical infrastructure supporting modern cloud computing requires an upgrade. Semiconductors that enable the development of GenAI models are in high demand, as is access to data centers and cloud computing services. Many of the companies tied to GenAI development (training) have appreciated meaningfully in response to the demand. Going forward, while we continue to see the potential upside of companies that provide the infrastructure for GenAI training, we believe the long-term opportunity for GenAI deployment (inference) could be even larger. We believe it is critical to distinguish the difference between the training and inference markets as we navigate the nascent GenAI theme.
GEN AI TRAINING
Training is the process where an AI model learns to identify and weigh attributes of input data, through a process of iterative guessing and correction, until it can consistently provide the correct output. The workload requires massive computing power. Training GPT-3, in 2020, required the computation of over 300 trillion math operations.1 The computing requirements to train GPT-4 (released in March 2023) are believed to be approximately 10 times greater than that of GPT-3.2 This level of computing is only made possible by the graphic processing unit (GPU), a specialized computer chip which found its first major use cases in video gaming applications. GPUs can perform several operations in parallel, unlike central processing units (CPUs), making them more efficient in running computations at scale. GPUs are foundational for generative AI training. As such, established companies designing and manufacturing GPUs could be well positioned in the GenAI training market.
INFERENCE, THE NEXT STAGE
Once a GenAI model is trained, the next phase is Inference, where the AI model is used to generate unique outputs based on new inputs. Unlike training, which requires iterative learning, inference focuses on applying the learned knowledge quickly and efficiently. It’s the difference between learning how to do new math problems in class (training) versus completing a worksheet of math problems that you know how to solve (inference). The computational workload during inference is generally less demanding than that of training. As such, the physical infrastructure required to support inference is different. While GPUs are instrumental in training AI models, they are not always the most efficient choice for running inference tasks. CPUs play a vital role, as will application-specific integrated circuits (ASICs). Networking hardware is also crucial for faster data transfer between servers running GenAI models and devices, as are power management chips for increasing energy efficiency. High performance solid-state drives (SSDs) will also be an increasingly important piece in the AI stack. SSDs provide faster data access and storage capabilities to reduce latency for AI inference. Going forward, we see opportunities in semiconductor and hardware firms tied to AI inference.
GenAI model development and deployment workloads: Training vs. Inference
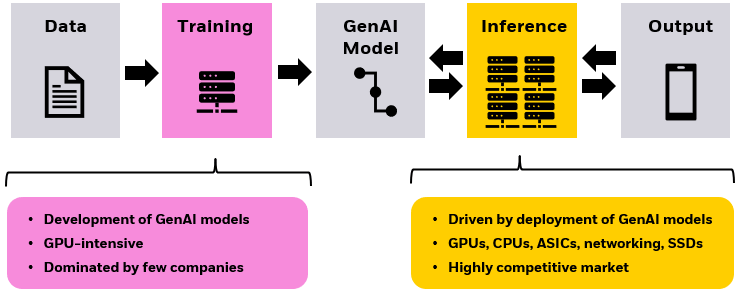
For illustrative purposes only. Subject to change.
Chart description: Flowchart highlighting the contrasting demands of two distinct phases in the Generative AI (GenAI) landscape. The training or development phase hinges on numerous GPUs, often controlled by a handful of major companies. In contrast, the subsequent inference phase is marked by intense market competition and a broader array of semiconductor options beyond GPUs.
OPPORTUNITIES IN GEN AI
As the use of generative AI grows and enterprises begin to implement the groundbreaking technology into daily work, smart phone apps, and more, we believe more focus will be placed on the infrastructure that enables inference. Relative to the market for the chips and hardware that enable training, we believe the inference market is more nuanced and more competitive, resulting in more potential opportunities for growth-oriented investors.
CONCLUSION
As various aspects of AI models and systems develop and emerge — across generative AI, machine learning, and natural-language processing — it is evident this is a mega force that will reshape multiple industries. People interested in investing in AI may consider exploring ETFs. Some ETFs offer exposure to the complete value chain of artificial intelligence, while others can dissect the traditional tech sector into smaller, essential industries such as semiconductors. Active ETFs, employing intensive research and active equity management, may also be primed to extract alpha from the evolving landscape. Given the growing significance of AI, owning these components could be beneficial for potential investors.